The Rise of Generative AI for Business
Aman kumar
11/26/20245 min read
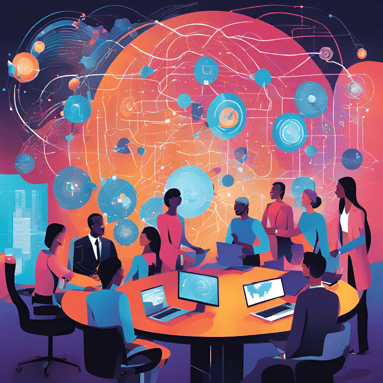
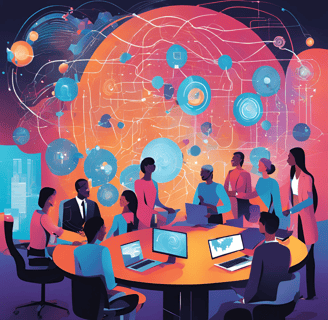
Artificial Intelligence has become one of the hottest topics of modern times, capturing imaginations and sparking debate globally. At the core of this revolution is Generative AI, a technology that creates entirely new content—from text and images to music and code—by interpreting massive amounts of data. While it may feel like magic, it's not. It's the result of decades of math, science, and innovation converging at this moment. The question isn’t if AI will change our world, but how—and that’s for all of us to determine.
AI: From Speculation to Reality
The idea that machines could "think" and process language isn't new. Alan Turing, often called the father of AI, proposed in 1950 that machines could simulate human intelligence. He predicted computers could master games like chess and even process natural language. That spark of inspiration eventually led to the “Big Bang” of AI in 1956: the Dartmouth Workshop, where the very term artificial intelligence was coined and the field formally took shape.
Back then, AI researchers outlined ambitious challenges, including neural networks and self-directed learning—some of which align with today’s breakthroughs. But this didn’t happen overnight. Progress came gradually as hardware, algorithms, and data capabilities evolved together.
In the 1950s, AI existed in theory, and computers relied on a mere handful of transistors. Fast forward to today, and the hardware landscape has transformed. GPUs now house over 100 billion transistors, working together to enable AI models. The journey from post-war theories to today’s generative AI isn’t just impressive—it's proof of what persistence in science can achieve.
What Powers Generative AI?
Generative AI stands on three pillars: model architecture, compute power, and data—commonly likened to a three-legged stool. Without any one component, the entire structure collapses. Let’s break it down:
Model Architecture: These are the algorithms that drive AI. Techniques like neural networks and transformers make modern generative AI possible.
Compute Power: Advances in hardware have dramatically cut the costs of processing power. GPUs and interconnected systems handle the intense demands of training large models.
Data: Models are only as good as the data they’re trained on. Generative AI learns from massive datasets, extracting patterns and relationships to create something entirely new.
Together, these elements form the backbone of technologies like large language models (LLMs). Trained with billions of data points, LLMs represent language in high-dimensional spaces, allowing them to predict word sequences, generate text, and much more.
Self-Supervised Learning: A Key Shift
Early AI depended on supervised learning, where humans labeled data for training. While effective, this process was slow and expensive, limiting its scalability. Everything changed in 2017 with the emergence of transformers and self-supervised learning.
In self-supervised learning, models train themselves on unlabeled data by guessing hidden or masked words—like solving a puzzle. This approach unlocked a way to scale learning without human intervention, leading to the development of foundation models.
These foundation models are adaptable, allowing industries to fine-tune them for specific tasks using their own curated data. For businesses, this means reducing deployment times while maintaining effectiveness.
How Generative AI Works
Generative AI isn’t just about crunching numbers; it’s about understanding relationships in data. For instance, when training on text, an AI model identifies patterns—like how words follow one another—and uses this knowledge to predict what comes next.
Imagine feeding an AI a prompt like, “Once upon a time.” The model predicts the most likely sequence of words to continue the story. By repeating this process across layers of neural networks, it generates something entirely new: a sentence, paragraph, or even an entire novel.
The same principles apply to other types of data. AI can connect pixels to create images, notes to compose music, or even chemical diagrams to assist in scientific discovery. If your data can be structured, chances are generative AI can work with it.
Generative AI in Business
Generative AI is no longer a niche tool; it’s becoming a cornerstone of business innovation. Its versatility allows applications across many fields. For example:
Human Resources: Automating repetitive tasks like résumé screening or crafting personalized onboarding materials.
Customer Service: Chatbots that provide accurate, human-like responses to common customer queries.
Cybersecurity: Detecting patterns in network traffic that may signal a threat.
Code Writing: Streamlining software development by generating or debugging code.
Application Modernization: Translating legacy systems into modern frameworks quickly and efficiently.
Generative AI’s reach extends beyond traditional language-focused tasks. AI interprets everything from user clicks on websites to sensor signals in industrial equipment as a kind of “language” it can analyze and optimize.
Challenges and Responsibilities
While the possibilities are endless, adopting AI comes with challenges, particularly around ethics and trust. Businesses must ensure their AI is both responsible and transparent. This means understanding the decisions AI makes and being able to explain its outputs.
One critical issue is bias. AI models trained on unfiltered or unethical data risk reinforcing harmful stereotypes or spreading misinformation. Ensuring datasets are accurate, relevant, and legally sourced is essential to creating fair systems.
AI also raises legitimate questions about its impact on jobs, privacy, and even society at large. Policymakers and business leaders must balance its transformative potential with safeguards that prevent misuse.
The Future of Generative AI
Generative AI is growing so rapidly that even short-term predictions are challenging. Models are evolving, open-source projects are proliferating, and new applications emerge daily. What’s clear is we’re heading toward a multimodal future.
Multimodal AI refers to systems capable of working across multiple types of data—think an AI that can analyze both audio and video simultaneously or combine written text with images. Such systems will unlock creative and practical applications we haven’t yet imagined.
The democratization of AI will also play a role. Open science and open-source initiatives can ensure AI’s development reflects diverse voices, not just those of a few tech giants. With transparency and collaboration, generative AI can benefit everyone.
How Businesses Can Prepare
As businesses look to adopt AI, planning is critical. Here are four actionable steps to consider:
Protect Your Data: Don’t outsource your competitive advantage. Your data is an asset—secure and own its usage.
Ensure Transparency: Make AI outputs explainable and foster trust among stakeholders.
Train Ethically: Use reliable, quality data free from bias or harmful content.
Stay in Control: Empower your teams with the tools and knowledge to oversee AI integration actively.
Generative AI isn’t just a tool you use—it’s a capability you shape. Taking an active role now ensures you’re not playing catch-up later.
Beyond Business: AI’s Role in Solving Major Challenges
Generative AI isn’t confined to improving processes or boosting productivity. It has the potential to tackle some of humanity’s greatest challenges. For instance:
Healthcare: Discovering new treatments or accelerating drug innovation.
Climate Action: Optimizing energy systems or finding breakthroughs in sustainable materials.
Scientific Research: Advancing fields like quantum physics or genomics.
While these examples are ambitious, they highlight the broader impacts AI could have if developed and deployed responsibly.
Conclusion
Generative AI is reshaping the way we think about business, technology, and the future. In just a short time, it has moved from novelty to necessity, with endless applications across industries. But with that power comes responsibility—and the chance to shape its role in our world.
Now is the time to explore how generative AI fits into your business strategy. Own your data, implement systems that are ethical and transparent, and empower your organization to be at the forefront of this technological transformation. AI isn’t just something we use—it’s something we shape together. Let’s make it count.